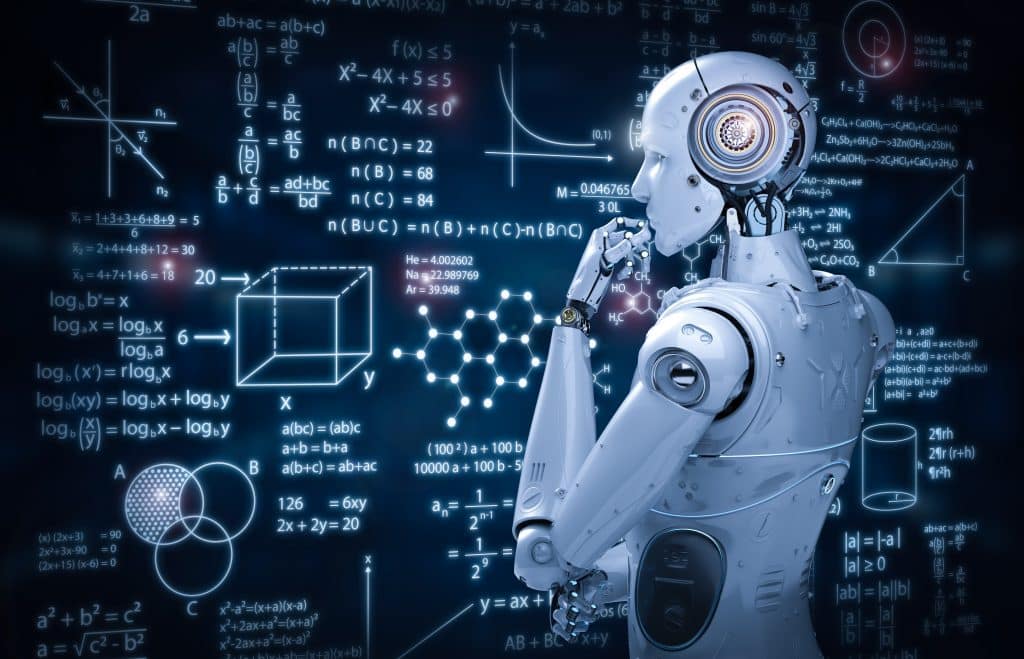
Machine learning is a subfield of artificial intelligence that focuses on the development of algorithms and statistical models that computers use to perform tasks without explicit instruction. Instead, these systems learn and improve from experience or by studying existing data. In essence, machine learning enables computers to find hidden insights and patterns and make decisions with minimal human intervention. Machine learning has been discovered to have a huge number of applications for the field of digital marketing services.
Why Machine Learning Is Effective in Digital Marketing
Machine learning offers a transformative edge to your marketing strategy by harnessing the power of data-driven insights and automation. Through sophisticated algorithms and data analysis, machine learning enhances customer segmentation, personalization, and predictive analytics, enabling you to craft precise and effective marketing campaigns. It optimizes advertising, streamlines content generation, and even empowers chatbots for round-the-clock customer support.
Additionally, machine learning aids in fraud detection, A/B testing, and competitive analysis, while also predicting customer lifetime value and preventing churn. By leveraging these capabilities, your marketing strategy can adapt to market trends, increase efficiency, and ultimately deliver a superior customer experience, all while optimizing costs and improving return on investment.
Ways to Use Machine Learning in Digital Marketing
Machine learning (ML) is extensively used in digital marketing across various domains. Here are some examples along with real-world business use cases:
Predictive Analytics
Netflix uses ML to predict which shows or movies users are likely to watch based on their viewing history. This drives content recommendations and keeps users engaged.
Customer Segmentation
Amazon segments customers based on their purchase history and browsing behavior. This allows them to send highly targeted product recommendations.
Personalization
Spotify uses ML to create personalized playlists for users based on their listening habits, enhancing the user experience and increasing user retention.
Content Recommendation
YouTube employs ML algorithms to recommend videos to users, keeping them engaged and increasing ad revenue through extended watch times.
Ad Targeting
Facebook’s ad platform uses ML to analyze user data and target ads to users who are most likely to engage with them, leading to higher conversion rates for advertisers.
Dynamic Pricing
Uber and Lyft use ML to adjust ride prices in real-time based on demand, traffic, and other factors, maximizing revenue.
Email Marketing
Grammarly personalizes email content by using ML to analyze user writing habits, which increases user engagement with their product.
Social Media Sentiment Analysis
Coca-Cola uses ML to analyze social media mentions and sentiment to gain insights into consumer opinions and adjust marketing strategies accordingly.
Chatbots
Sephora uses chatbots on their website to provide product recommendations and answer customer questions, improving user experience and driving sales.
Search Engine Optimization (SEO)
Moz uses ML to provide recommendations for optimizing website content, helping businesses improve their search engine rankings.
Fraud Detection
PayPal employs ML to detect fraudulent transactions by analyzing transaction patterns and user behavior, protecting both the company and its customers.
Voice Search Optimization
Domino’s Pizza uses voice search optimization to allow customers to place orders through voice assistants like Amazon Alexa and Google Assistant.
Content Generation
The Washington Post uses ML to automatically generate short articles and reports on topics like sports scores and election results.
Lead Scoring
Salesforce’s Einstein Lead Scoring uses ML to rank leads based on their likelihood to convert, enabling sales teams to focus on the most promising prospects.
Attribution Modeling
Google Analytics uses ML to provide data-driven attribution models that help businesses understand the impact of each marketing channel on conversions.
These examples showcase how machine learning is applied in various facets of digital marketing to optimize processes, improve customer experiences, and drive business growth. Many companies, large and small, are leveraging these techniques to gain a competitive edge in the digital landscape.
Implementing Machine Learning in Digital Marketing
Implementing machine learning in digital marketing involves a series of steps. Here are 10 key steps to guide you through the process:
Define Clear Objectives
Begin by clearly defining your marketing goals and objectives. Understand what specific challenges or opportunities you aim to address with machine learning. For example, you might want to improve ad targeting, increase customer retention, or optimize content recommendations.
Data Collection and Preparation
Gather relevant data from various sources such as customer interactions, website analytics, CRM systems, and social media. Ensure that the data is comprehensive, accurate, and properly formatted. Data preprocessing, cleaning, and feature engineering are critical steps to make the data suitable for machine learning.
Select Appropriate Algorithms
Choose the machine learning algorithms that align with your objectives and dataset characteristics. Common algorithms for digital marketing include regression, classification, clustering, and recommendation algorithms. Select the right tools and libraries (e.g., scikit-learn, TensorFlow, or PyTorch) for implementing these algorithms.
Data Splitting and Model Training
Split your dataset into training, validation, and test sets. Train your machine learning models on the training data and validate them on the validation set to fine-tune parameters and optimize performance. The test set is used for final evaluation.
Feature Selection and Engineering
Identify and select the most relevant features (variables) from your dataset to improve model accuracy and reduce overfitting. Additionally, engineer new features that may provide valuable insights to the model.
Model Evaluation and Optimization
Assess the performance of your machine learning models using appropriate metrics (e.g., accuracy, F1 score, AUC-ROC). Optimize your models through techniques like hyperparameter tuning, cross-validation, and ensemble methods to achieve the best results.
Integration with Marketing Platforms
Integrate your trained machine learning models with your marketing platforms and systems. This could involve integrating with advertising platforms, content management systems, email marketing tools, or customer relationship management (CRM) systems.
Automation and Personalization
Automate marketing tasks using machine learning insights. For instance, automate email campaigns based on customer behavior, personalize website content in real-time, or optimize ad bids and targeting.
Monitoring and Maintenance
Continuously monitor the performance of your machine learning models in a production environment. Set up alerting systems to detect anomalies or deteriorating model performance. Regularly retrain and update models as new data becomes available.
Compliance and Data Privacy
Ensure that your machine learning implementation complies with data privacy regulations such as GDPR or CCPA. Be transparent with customers about how their data is being used and obtain necessary consents.
The implementation of machine learning in digital marketing is an iterative process. Regularly assess the impact of your machine learning initiatives on your marketing efforts and make adjustments as needed to achieve your objectives. Collaboration between marketing and data science teams is essential for successful implementation and ongoing optimization.